AI-based Postoperative Infection Prediction: External Validation and Local Recalibration of PERISCOPE
Author(s):
Siri van der Meijden; Anna van Boekel; Mark G. J. de Boer; Rob G. H. H. Nelissen; Bart F. Geerts; Dieter Mesotten; Ewout W. Steyerberg; Sesmu M.S. Arbous; Harry Van Goor
Background: To improve postoperative infection management, we developed PERISCOPE, an Artificial Intelligence (AI) based decision support tool. Multicenter external validations of AI models are rare and often reveal poor generalizability, exemplified by a widely implemented sepsis prediction model with low predictive performance. Hence, we conducted a multicenter study to explore external validity and the need for recalibrating PERISCOPE with local data before clinical implementation.
Hypothesis: Local recalibrated versions of PERISCOPE improve model performance and therefore enhance potential clinical utility.
Methods: An AI model was developed to predict all types of postoperative infections within 30 days of surgery in hospital A (LUMC, the Netherlands). Postoperative infections were defined as any infection registered and/or requiring pharmacological and/or interventional treatment. Hospital A’s model was externally validated on retrospective data from hospital B (Radboud UMC, the Netherlands) and hospital C (ZOL Genk, Belgium). All three datasets were divided into a development dataset and a test dataset that included the two most recent years of data. Models were recalibrated, including refitting of the model’s weights, on hospital B’s and C’s development dataset. The three local 30-day models were evaluated on each hospital’s temporal test dataset by assessing the area under the receiver operating characteristic curve (AUROC).
Results: 30-day infection rates were respectively 14%, 14% and 4% in hospitals A, B and C’s test dataset. Locally recalibrated models achieved the highest discriminative model performance in each site (Table 1), with AUROCs between 0.82 and 0.91, overall indicating good model performance.
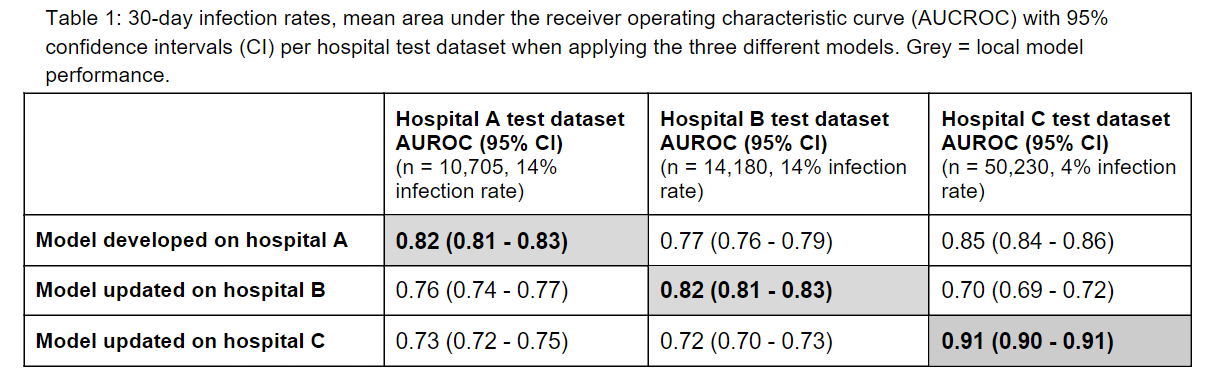
Conclusions: Local recalibrated models achieved higher discriminative performance for predicting postoperative infections within 30 days of surgery, resulting in high predictive accuracy among sites. Further studies may elucidate the reasons for differential predictive performance, including heterogeneity between hospitals in patient and surgery characteristics, incidence rates, clinical protocols, and professionals involved.